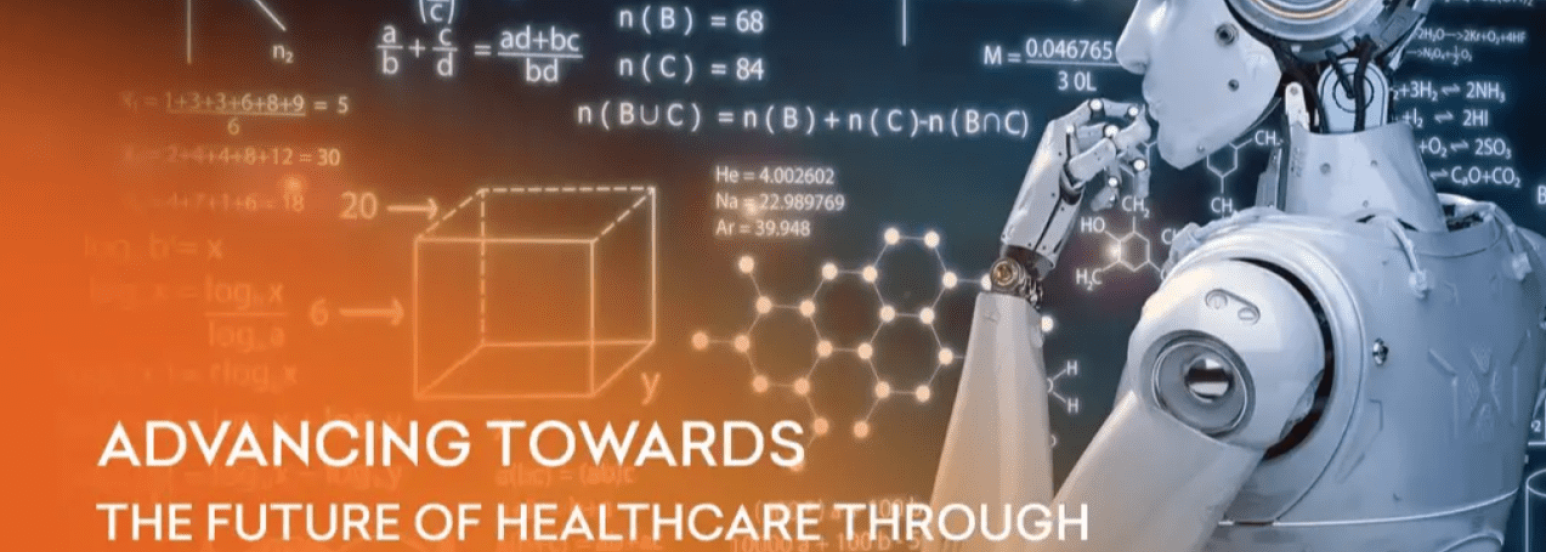
In recent years, the healthcare industry has been increasingly turning to machine learning and data analytics to improve patient outcomes, streamline operations, and reduce costs. From predicting patient outcomes to identifying trends in population health, the potential uses for these technologies are vast and varied.
Predictive modeling and diagnosis
One of the most promising applications of machine learning in healthcare is the ability to predict patient outcomes based on data from past patients. By analyzing large datasets of patient records, machine learning algorithms can identify patterns and trends that can help doctors make more accurate diagnoses and treatment recommendations. This can be especially useful for rare or complex conditions, where a lack of data can make it difficult to make informed decisions.
Population health management
Machine learning can also be used to identify trends in population health, such as the prevalence of certain diseases in certain populations. This can help healthcare providers target their efforts more effectively, and allow them to intervene early to prevent the spread of diseases. Additionally, data analytics can be used to identify patterns in the factors that contribute to poor health outcomes, such as diet, exercise, and stress, which can inform public health campaigns and policies.
Efficiency improvements
In addition to improving patient care, machine learning and data analytics can also be used to streamline healthcare operations. For example, data analytics can be used to identify bottlenecks in the healthcare system, such as long wait times or inefficiencies in the scheduling process. By identifying these issues and implementing solutions, healthcare providers can improve the efficiency of their operations, which can ultimately lead to better patient experiences.
Challenges and considerations
While the potential benefits of machine learning and data analytics in healthcare are clear, there are also a number of challenges and considerations to keep in mind. One of the main challenges is ensuring the accuracy and reliability of the data being used. With machine learning, the quality of the data plays a key role in the accuracy of the predictions and insights generated. Additionally, there are also concerns about data privacy and security, as well as the potential for bias in the algorithms if the data is not representative of the population as a whole.
Despite these challenges, the potential for machine learning and data analytics in healthcare is vast, and as these technologies continue to evolve, it's likely that we will see even more innovative applications in the future.